-
Posts
5,559 -
Joined
-
Last visited
-
Days Won
864
Lurker last won the day on July 18
Lurker had the most liked content!
About Lurker
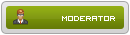
- Birthday 02/13/1983
Profile Information
-
Gender
Male
-
Location
INDONESIA
-
Interests
GIS and Remote Sensing
Recent Profile Visitors
The recent visitors block is disabled and is not being shown to other users.
Lurker's Achievements
-
When two Finnair planes flying into Estonia recently had to divert in quick succession and return to Helsinki, the cause wasn’t a mechanical failure or inclement weather—it was GPS denial. GPS denial is the deliberate interference of the navigation signals used and relied on by commercial aircraft. It’s not a new phenomenon: The International Air Transport Association (IATA) has long provided maps of regions where GPS was routinely unavailable or untrusted. However, concern is growing rapidly as conflict spreads across Europe, the Middle East, and Asia, and GPS jamming and spoofing become weapons of economic and strategic influence. Several adversarial nations have been known to use false (spoofed) GPS signals to interfere with air transit, shipping, and trade, or to disrupt military logistics in conflict zones. And recent discussions of anti-satellite weapons renewed fears of deliberate actions designed to wreak economic havoc by knocking out GPS. GPS has become so ubiquitous in our daily lives that we hardly think about what happens when it’s not available A GPS outage would result in many online services becoming unavailable (these rely on GPS-based network synchronization), failure of in-vehicle Satnav, and no location-based services on your mobile phone. Analyses in the U.S. and U.K. have both identified the temporary economic cost of an outage at approximately $1 billion per day—but the strategic impacts can be even more significant, especially in a conflict. The saying is that infantry wins battles, but logistics wins wars. It’s almost unimaginable to operate military logistics supply chains without GPS given the heavy reliance on synchronized communications networks, general command and control, and vehicle and materiel positioning and tracking. All of these centrally rely on GPS and all are vulnerable to disruption. Most large military and commercial ships and aircraft carry special GPS backups for navigation because there was, in fact, a time before GPS GPS is not available in all settings—underground, underwater, or at high latitudes. The GPS alternatives rely on signals that can be measured locally (for instance, motion or magnetic fields as used in a compass), so a vessel can navigate even when GPS is unavailable or untrusted. For example, inertial navigation uses special accelerometers that measure vehicle motion, much like the ones that help your mobile phone reorient when you rotate it. Measuring how the vehicle is moving and using Newton’s laws allows you to calculate your likely position after some time. Other “alt-PNT” approaches leverage measurements of magnetic and gravitational fields to help navigate against a known map of these variations near the Earth’s surface. Plus, ultrastable locally deployed clocks can ensure communications networks remain synchronized during GPS outages (comms networks typically rely on GPS timing signals to remain synchronized). Nonetheless, we rely on GPS because it’s simply much better than the backups. Focusing specifically on positioning and navigation, achieving good performance with conventional alternatives typically requires you to significantly increase system complexity, size, and cost, limiting deployment options on smaller vehicles. Those alternative approaches to navigation are also unfortunately prone to errors due to the instability of the measurement equipment in use—signals just gradually change over time, with varying environmental conditions, or with system age. We keep today’s alternatives in use to provide a backstop for critical military and commercial applications, but the search is on for something significantly better than what’s currently available. That something looks to be quantum-assured navigation, powered by quantum sensors. Quantum sensors rely on the laws of nature to access signatures that were previously out of reach, delivering both extreme sensitivity and stability As a result, quantum-assured navigation can deliver defense against GPS outages and enable transformational new missions. The most advanced quantum-assured navigation systems combine multiple sensors, each picking up unique environmental signals relevant to navigation, much the way autonomous vehicles combine lidar, cameras, ultrasonic detectors, and more to deliver the best performance. This starts with a new generation of improved quantum inertial navigation, but quantum sensing allows us to go further by accessing new signals that were previously largely inaccessible in real-world settings. While it may be surprising, Earth’s gravity and magnetic fields are not constant everywhere on the planet’s surface. We have maps of tiny variations in these quantities that have long been used for minerals prospecting and even underground water monitoring. We can now repurpose these maps for navigation. We’re building a new generation of quantum gravimeters, magnetometers, and accelerometers—powered by the quantum properties of atoms to be sensitive and compact enough to measure these signals on real vehicles. The biggest improvements come from enhanced stability. Atoms and subatomic particles don’t change, age, or degrade—their behavior is always the same. That’s something we are now primed to exploit. Using a quantum-assured navigation system, a vehicle may be able to position itself precisely even when GPS is not available for very long periods. Not simply hours or days as is achievable with the best military systems today, but weeks or months. In quantum sensing, we have already achieved quantum advantage—when a quantum solution decidedly beats its conventional counterparts. The task at hand is now to take these systems out of the lab and into the field in order to deliver true strategic advantage. That’s no mean feat. Real platforms are subject to interference, harsh conditions, and vibrations that conspire to erase the benefits we know quantum sensors can provide. In recent cutting-edge research, new AI-powered software can be used to deliver the robustness needed to put quantum sensors onto real moving platforms. The right software can keep the systems functional even when they’re being shaken and subjected to interference on ships and aircraft. To prevent a repeat of the Finnair event, real quantum navigation systems are now starting to undergo field testing. Our peers at Vector Atomic recently ran maritime trials of a new quantum optical clock. The University of Birmingham published measurements with a portable gravity gradiometer in the field. At Q-CTRL, we recently announced the world’s first maritime trial of a mobile quantum dual gravimeter for gravity map matching at a conference in London. My team is excited to now work with Airbus, which is investigating software-ruggedized quantum sensors to provide the next generation of GPS backup on commercial aircraft. Our full quantum navigation solutions are about to commence flight safety testing with the first flights later in the year, following multiple maritime and terrestrial trials. With a new generation of quantum sensors in the field, we’ll be able to ensure the economy keeps functioning even in the event of a GPS outage. From autonomous vehicles to major shipping companies and commercial aviation, quantum-assured navigation is the essential ingredient in providing resilience for our entire technology-driven economy.
-
A Falcon 9 successfully launched an Earth science mission for Europe and Japan May 28 as part of the European Space Agency’s ongoing, if temporary, reliance on SpaceX for space access. The Falcon 9 lifted off from Vandenberg Space Force Base in California at 6:20 p.m. Eastern. The payload, the Earth Cloud Aerosol and Radiation Explorer (EarthCARE) spacecraft, separated from the upper stage about 10 minutes after liftoff. Simonetta Cheli, director of Earth observation programs at ESA, said in a post-launch interview that controllers were in contact with the spacecraft. “It is all nominal and on track.” Spacecraft controllers will spend the weeks and months ahead checking out the spacecraft’s instruments and calibrating them, she said. That will allow the first release of science data from EarthCARE around the end of this year or early next year. EarthCARE is an 800-million-euro ($870 million) ESA-led mission to study clouds and aerosols in the atmosphere. The spacecraft carries four instruments, including a cloud profiling radar provided by the Japanese space agency JAXA at a cost of 8.3 billion yen ($53 million). JAXA dubbed the spacecraft Hakuryu or “White Dragon” because of the spacecraft’s appearance. The 2,200-kilogram spacecraft, flying in a sun-synchronous orbit at an altitude of 393 kilometers, will collect data on clouds and aerosols in the atmosphere, along with imagery and measurements of reflected sunlight and radiated heat. That information will be used for atmospheric science, including climate and weather models. “EarthCARE is there to study the effect of clouds and aerosols on the thermal balance of the Earth,” said Dirk Bernaerts, ESA’s EarthCARE project manager, at a pre-launch briefing May 21. “It’s very important to observe them all together at the same location at the same time. That is what is unique about this spacecraft.” Other spacecraft make similar measurements, including NASA’s Plankton, Aerosol, Cloud, ocean Ecosystem (PACE) spacecraft launched in February. “The observation techniques are different,” he said. “We observe the same thing but observe slightly different aspects of the clouds and aerosols.” He added that EarthCARE would use PACE data to help with calibration and validation of its observations. Development of EarthCARE took about two decades and resulted in cost growth that Cheli estimated at the pre-launch briefing to be 30%. Maximilian Sauer, EarthCARE project manager at prime contractor Airbus, said several factors contributed to the delays and overruns, including technical issues with the instruments as well as effects of the pandemic. One lesson learned from EarthCARE, Cheli said in the post-launch interview, was the need for “strict management” of the project, which she said suffered from challenges of coordinating work between agencies and companies. The mission also underscored the importance of strong support from member states as it worked to overcome problems, she added. Another factor in EarthCARE’s delay was a change in launch vehicles. EarthCARE was originally slated to go on a Soyuz rocket but ESA lost access to the vehicle after Russia’s invasion of Ukraine. The mission was first moved to Europe’s Vega C, but ESA decided last June to launch it instead on a Falcon 9, citing delaying in returning that rocket to flight as well as modifications to the rocket’s payload fairing that would have been needed to accommodate EarthCARE. Technically, the shift in launch vehicles was not a major problem for the mission. “Throughout the changes in the launchers we did not have to change the design of the spacecraft,” said Bernaerts. He said that, during environmental tests, engineers put the spacecraft through conditions simulating different launch vehicles to prepare for the potential of changing vehicles. “From the moment we knew that Soyuz was not available, we have been looking at how stringently we could test the spacecraft to envelope other candidate launchers. That’s what we did and that worked out in the end.” EarthCARE is the second ESA-led mission to launch on a Falcon 9, after the Euclid space telescope last July. Another Falcon 9 will launch ESA’s Hera asteroid mission this fall. “We had a good experience with Euclid last year,” said Josef Aschbacher, ESA director general, in a post-launch interview. “Our teams and the SpaceX teams are working together very well.” The use of the Falcon 9 is a stopgap until Ariane 6 enters services, with a first launch now scheduled for the first half of July, and Vega C returns to flight at the end of the year. “I hear lots of questions about why we’re launching with Falcon and not with Ariane, and it’s really good to see the Ariane 6 inaugural flight coming closer,” he said. Those involved with the mission were simply happy to finally get the spacecraft into orbit. “There is a feeling of relief and happiness,” Cheli said after the launch. “This is an emotional roller coaster,” said Thorsten Fehr, EarthCARE mission scientist at ESA, on the agency webcast of the launch shortly after payload separation. “This is one of the greatest moments in my professional life ever.”
-
Maker Ilia Ovsiannikov is working on a friendly do-it-yourself robot kit — and as part of that work has released a library to make it easier to use a range of lidar sensors in your Arduino sketches. "I have combined support for various spinning lidar/LDS sensors into an Arduino LDS library with a single platform API [Application Programming Interface]," Ovsiannikov explains. "You can install this library from the Arduino Library Manager GUI. Why support many lidar/LDS sensors? The reason is to make the hardware — supported by [the] Kaia.ai platform — affordable to as many prospective users as possible. Some of the sensors [suported] are sold as used robot vacuum cleaner spare parts and cost as low as $16 or so (including shipping)." The library delivers support for a broad range of lidar sensors from a unified API, Ovsiannikov explains, meaning it's not only possible to get started quickly but to switch sensors mid-project — should existing sensors become unavailable, or pricing shift to favor a different model. It also adds a few neat features of its own, including pulse-width modulation (PWM) control of lidar motors that lack their own control system, using optional adapter boards. While the library is usable standalone, and even to perform real-time angle and distance computation directly on an Arduino microcontroller, Ovsiannikov has also published a companion package to tie it in to the Robot Operating System 2 (ROS2). "[The] Kaia.ai robot firmware forwards LDS raw data — obtained from the Arduino LDS library — to a PC running ROS2 and micro-ROS," he explains. "The ROS2 PC kaiaai_telemetry package receives the raw LDS data, decodes that data and publishes it to the ROS2 /scan topic." More information on the library is available in Ovsiannikov's blog post, while the library itself is available on GitHub under the permissive Apache 2.0 license. more information: https://kaia.ai/blog/arduino-lidar-library/ https://github.com/kaiaai/LDS
-
cool stuff
-
has been discuss in their forum https://www.agisoft.com/forum/index.php?topic=2420.0
-
Traditionally, surveying relied heavily on manual measurements and ground-based techniques, which were time-consuming, labour-intensive and often limited in scope. With the somewhat recent emergence of photogrammetry, surveying professionals gained access to powerful tools that streamline processes, improve accuracy and unlock new possibilities in data collection and analysis. How videogrammetry works Videogrammetry is effectively an extension of photogrammetry; the mechanics are grounded in similar principles. Photogrammetry involves deriving accurate three-dimensional information from two-dimensional images by analysing their geometric properties and spatial relationships. The core of videogrammetry is to separate the video footage into images with regard to sufficient overlap and image quality. The subsequent workflow is almost the same as in photogrammetry. The quality of the output depends heavily on image resolution, frames per second (FPS) and stabilization. Integration with hardware and software Central to videogrammetry is the camera system used to capture video footage along with any supporting GPS devices used. Ground-based videogrammetry may utilize smartphone cameras or handheld digital cameras with video capabilities. While many smartphones and digital cameras have built-in GPS capabilities that geotag photographs with location data, their accuracy is at best 2.5m which is not always optimal for professional surveying. For this reason, when using a smartphone camera, many surveyors opt for an external real-time kinematic (RTK) antenna with 2cm accuracy. Attaching it to their smartphones enables them to receive correction data from a local NTRIP provider. This is a far more convenient option than placing and measuring ground control points (GCPs) on the terrain in combination with a GNSS device. Some of the well-known smartphone RTK products on the market today include REDCatch’s SmartphoneRTK, ArduSimple’s ZED-F9P RTK receiver, and Pix4D’s ViDoc RTK. It is important to note that while most smartphones can geotag photographs, not all can geotag video footage. Moreover, those smartphones that can geotag video can only geotag the first frame of the video. For this reason, users may require additional apps (e.g. PIX4Dcatch: 3D scanner) that can embed location data into the video’s metadata so that it can be used for surveying and mapping purposes. While non-geotagged videos can still be used for 3D model creation in various software solutions, it is recommended to opt for an RTK receiver as a minimum prerequisite for professional applications. At 3Dsurvey, utilization of Google’s Pixel 7a smartphone paired with REDcatch’s external RTK is the preferred option. Later this year, 3Dsurvey is set to release a ScanApp that it has developed to embed RTK correction data into video file metadata, enabling automatic georeferencing for videogrammetry projects. Examples of videogrammetry project approaches Non-geotagged (using any smartphone or camera) This can be ideal for the 3D documentation of cultural heritage projects. However, this approach lacks the spatial accuracy necessary for tasks such as outdoor mapping or infrastructure monitoring, which demand precise georeferencing. Accurately geotagged using external RTK (using a smartphone) Accurate geotagging using a smartphone equipped with an external RTK GNSS receiver ensures that the resulting 3D models maintain high spatial fidelity. Therefore, this approach is suitable for applications such as land surveying and small-scale construction monitoring projects where precise positioning is crucial. Examples include mapping manholes, pipes, low-level material piles, dig sites and cables for telecommunication or electricity. Within a larger photogrammetry/Lidar project For situations demanding the highest level of accuracy, videogrammetry can fill a gap or add another perspective to the aerial dataset obtained using other technologies, such as ground-level videogrammetry in combination with aerial Lidar (which lacks oblique views). Videogrammetry can also prove invaluable on site while drone mapping, such as when trees obstruct the flight path or if the project requires capturing details facing upwards. Similar to drone workflows, strategically placed and precisely measured GCPs can significantly improve the overall precision of the generated 3D model. Since videogrammetry usually involves capturing data from low angles, consider using AprilTag targets for superior oblique detection. Challenges and considerations Videogrammetry offers immense potential for various applications, yet its implementation comes with a set of challenges. Filming excessive footage can result in software inaccuracies, leading to duplicate surfaces in the 3D model. Therefore, it is important to carefully consider the path taken when filming. Some areas may be challenging to film, but if those areas are not captured in the video, they cannot be included when reconstructing the 3D model. Filming while navigating through obstacles, especially on construction sites, requires caution and precision on the part of the user. This sometimes gets in the way of creating a perfect video. Weather conditions such as puddles and sunlight can affect data accuracy by creating reflective surfaces and casting shadows, respectively. Filming areas obstructed by roofs, trees or walls can degrade the RTK signal, leading to inaccuracies in the final model. Tips for accurate data capture The quality of the output depends on a number of factors, including how the data is captured. The following basic principles can help users to obtain the necessary coordinates when filming so that the 3D model will be as realistic as possible: Move slowly and steadily: To obtain sharp images, maintain slow and smooth movements. This is especially crucial in poor light conditions when the shutter speed is low and video frames are more susceptible to blur. Rotate slowly and move while turning: Just like when towing a trailer, it is necessary to move back and forth rather than trying to turn on the spot. Don’t ‘wall paint’ when scanning vertical surfaces: Standing in one place while tilting the device up and down will generate a lot of images, but they will all have the same coordinates. Instead, move in a lateral direction while recording at different heights. Film in connected/closed loops: Try to ensure that the filming ends precisely back at the starting point. Advantages of videogrammetry Videogrammetry offers significant advantages in surveying, particularly when smartphones are leveraged as data capture devices. The portability and convenience of smartphones enables swift, efficient and accessible data collection in a wide range of situations, making it possible to document areas that are either small, rapidly changing, or require close-up details in hard-to-reach places. Moreover, unlike traditional methods requiring specialized equipment and expertise, smartphone videogrammetry empowers more professionals to capture and reconstruct 3D data. The standout feature of videogrammetry is that it eliminates overlap concerns, since the video is continuously shot at approximately 30FPS. This accessibility paves the way for even broader surveying applications. Integrating videogrammetry into a data collection toolkit promises to accelerate project timelines, streamline workflows and above all improve responsiveness, since a smartphone is always on site. This makes videogrammetry a cost-effective solution for surveying tasks. Videogrammetry in practice These three case studies illustrate the practical application of videogrammetry in various situations, ranging from a simple scenario to a mid-sized construction site. A Pixel smartphone with RTK and the 3Dsurvey ScanApp were used in all cases. 1. Pile volume calculation Photogrammetry is commonly used to calculate the volume of material piles, but the pre-flight preparation and planning can be time-consuming. Moreover, drones are bulky and less portable than a smartphone. Therefore, videogrammetry – with a handheld RTK antenna connected to a smart phone – can offer a much faster and simpler alternative. In this project to capture a small pile of material, the smartphone was simply held up high, tilted down and encircled the heap. During a five-minute site visit, a 75-second video was recorded, from which 152 frames were extracted. The processing time amounted to 30 minutes. 2. Preserving cultural heritage Archaeological excavation sites and statues that require 3D documentation are often located in crowded urban areas which may be subject to strict drone regulations. Some culturally significant items may be located indoors, such as in museums, where photogrammetry is challenging. Moreover, using ground surveying equipment like laser scanners requires highly technical knowledge. This can be an expensive option and therefore unsuitable for such projects. In a project to capture a complete and accurate 3D scan of a dragon statue, a total of 20 minutes were spent on site. 226 frames were extracted from 113 seconds of video. The subsequent processing time was one hour. 3. Underground infrastructure project Videogrammetry can be successfully used in the context of underground construction and engineering projects, such as when laying pipes into a trench. Compared with documenting the site with traditional equipment, using a smartphone equipped with RTK technology makes the process of documenting remarkably efficient. Just as with drone photogrammetry, multiple mappings can be performed to track progress. As another advantage, videogrammetry makes it possible to get really close and record details that may be hidden from the top-down aerial view. In support of a construction and engineering project for the installation of underground fuel tanks, videogrammetry was used to document and extract the exact measurements, monitor the width and the depth, calculate the volume and extract profile lines. A 15-minute site visit was sufficient to record 87 seconds of video, leading to 175 extracted frames. The processing time amounted to 45 minutes. Conclusion While it should be pointed out that it is not a replacement for established surveying techniques like photogrammetry and laser scans, videogrammetry has emerged as a valuable addition to the surveyor’s toolkit. Overall, this technology offers professionals significant gains in convenience, efficiency and flexibility, because surveyors can capture data using just a smartphone. This allows for faster and more accessible data capture across versatile situations, and provides cost-effective solutions adaptable to specific needs thanks to integrating seamlessly with existing surveying workflows. While filming with a smartphone currently has its limitations that present challenges, continuous advancements in hardware, software and best practices are steadily improving the accuracy and reliability of data collection. This ongoing evolution means that videogrammetry has the potential to contribute to better-informed decision-making and become an indispensable tool for the modern construction professional. source : gim-international
-
something like this? python - Polygon clustering - Geographic Information Systems Stack Exchange
-
what version of qgis do you use right now?
-
is it the error occured in all the historical data you've check?
-
my previous post address your first question actually 😁 I saw that you already got the the equation for the band value and the depth, so it should be okay you directly apply it to get depth value
-
Researchers at the University of Science and Technology of China (USTC) have developed a compact and lightweight single-photon LiDAR system that can be deployed in the air to generate high-resolution three-dimensional images with a low-power laser. The technology could be used for terrain mapping, environmental monitoring, and object identification, according to a press release. LiDAR, which stands for Light Detection And Ranging, is extensively used to determine geospatial information. The system uses light emitted by pulse lasers and measures the time taken by the reflected light to be received to determine the range, creating digital twins of objects and examining the surface of the earth. A common application of the system has been to help autonomous driving systems or airborne drones determine their environments. However, this requires an extended setup of LIDAR sensors, which is power-intensive. To minimize such sensors’ energy consumption, USTC researchers devised a single-photon lidar system and tested it in an airborne configuration. The single-photon lidar The single-photon lidar system is made possible by detection systems that can measure the small amounts of light given out by the laser when it is reflected. The researchers had to shrink the entire LiDAR system to develop it. It works like a regular LiDAR system when sending light pulses toward its targets. To capture the small amounts of light reflected, the team used highly sensitive detectors called single-photon avalanche diode (SPAD) arrays, which can detect single photons. To reduce the overall system size, the team also used small telescopes with an optical aperture of 47 mm as receiving optics. The time-of-flight of the photons makes it possible to determine the distance to the ground, and advanced computer algorithms help generate detailed three-dimensional images of the terrain from the sensor. “A key part of the new system is the special scanning mirrors that perform continuous fine scanning, capturing sub-pixel information of the ground targets,” said Feihu Xu, a member of the research team at USTC. “Also, a new photon-efficient computational algorithm extracts this sub-pixel information from a small number of raw photon detections, enabling the reconstruction of super-resolution 3D images despite the challenges posed by weak signals and strong solar noise.” Testing in real-world scenario To validate the new system, the researchers conducted daytime tests onboard a small airplane in Yiwu City, Zhejiang Province. In pre-flight ground tests, the LiDAR demonstrated a resolution of nearly six inches (15 cm) from nearly a mile (1.5 km). The team then implemented sub-pixel scanning and 3D deconvolution and found the resolution improved to 2.3 inches (six cm) from the same distance. “We were able to incorporate recent technology developments into a system that, in comparison to other state-of-the-art airborne LiDAR systems, employs the lowest laser power and the smallest optical aperture while still maintaining good performance in detection range and imaging resolution,” added Xu. The team is now working to improve the system’s performance and integration so that a small satellite can be equipped with such tech in the future. “Ultimately, our work has the potential to enhance our understanding of the world around us and contribute to a more sustainable and informed future for all,” Xu said in the press release. “For example, our system could be deployed on drones or small satellites to monitor changes in forest landscapes, such as deforestation or other impacts on forest health. It could also be used after earthquakes to generate 3D terrain maps that could help assess the extent of damage and guide rescue teams, potentially saving lives.”
-
comparison of shallow water depth algorithm https://ejournal2.undip.ac.id/index.php/jkt/article/view/16050
-
Multimodal machine learning models have been surging in popularity, marking a significant evolution in artificial intelligence (AI) research and development. These models, capable of processing and integrating data from multiple modalities such as text, images, and audio, are of great importance due to their ability to tackle complex real-world problems that traditional unimodal models struggle with. The fusion of diverse data types enables these models to extract richer insights, enhance decision-making processes, and ultimately drive innovation. Among the burgeoning applications of multimodal machine learning, Visual Question Answering (VQA) models have emerged as particularly noteworthy. VQA models possess the capability to comprehend both images and accompanying textual queries, providing answers or relevant information based on the content of the visual input. This capability opens up avenues for interactive systems, enabling users to engage with AI in a more intuitive and natural manner. However, despite their immense potential, the deployment of VQA models, especially in critical scenarios such as disaster recovery efforts, presents unique challenges. In situations where internet connectivity is unreliable or unavailable, deploying these models on tiny hardware platforms becomes essential. Yet the deep neural networks that power VQA models demand substantial computational resources, rendering traditional edge computing hardware solutions impractical. Inspired by optimizations that have enabled powerful unimodal models to run on tinyML hardware, a team led by researchers at the University of Maryland has developed a novel multimodal model called TinyVQA that allows extremely resource-limited hardware to run VQA models. Using some clever techniques, the researchers were able to compress the model to the point that it could run inferences in a few tens of milliseconds on a common low-power processor found onboard a drone. In spite of this substantial compression, the model was able to maintain acceptable levels of accuracy. To achieve this goal, the team first created a deep learning VQA model that is similar to other state of the art algorithms that have been previously described. This model was far too large to use for tinyML applications, but it contained a wealth of knowledge. Accordingly, the model was used as a teacher for a smaller student model. This practice, called knowledge distillation, captures much of the important associations found in the teacher model, and encodes them in a more compact form in the student model. In addition to having fewer layers and fewer parameters, the student model also made use of 8-bit quantization. This reduces both the memory footprint and the amount of computational resources that are required when running inferences. Another optimization involved swapping regular convolution layers out in favor of depthwise separable convolution layers — this further reduced model size while having a minimal impact on accuracy. Having designed and trained TinyVQA, the researchers evaluated it by using the FloodNet-VQA dataset. This dataset contains thousands of images of flooded areas captured by a drone after a major storm. Questions were asked about the images to determine how well the model understood the scenes. The teacher model, which weighs in at 479 megabytes, was found to have an accuracy of 81 percent. The much smaller TinyVQA model, only 339 kilobytes in size, achieved a very impressive 79.5 percent accuracy. Despite being over 1,000 times smaller, TinyVQA only lost 1.5 percent accuracy on average — not a bad trade-off at all! In a practical trial of the system, the model was deployed on the GAP8 microprocessor onboard a Crazyflie 2.0 drone. With inference times averaging 56 milliseconds on this platform, it was demonstrated that TinyVQA could realistically be used to assist first responders in emergency situations. And of course, many other opportunities to build autonomous, intelligent systems could also be enabled by this technology. source: hackster.io
-
A new machine learning system can create height maps of urban environments from a single synthetic aperture radar (SAR) image, potentially accelerating disaster planning and response. Aerospace engineers at the University of the Bundeswehr in Munich claim their SAR2Height framework is the first to provide complete—if not perfect—three-dimensional city maps from a single SAR satellite. When an earthquake devastates a city, information can be in short supply. With basic services disrupted, it can difficult to assess how much damage occurred or where the need for humanitarian aid is greatest. Aerial surveys using laser ranging lidar systems provide the gold standard for 3D mapping, but such systems are expensive to buy and operate, even without the added logistical difficulties of a major disaster. Remote sensing is another option but optical satellite images are next to useless if the area is obscured by clouds or smoke. Synthetic aperture radar, on the other hand, works day or night, whatever the weather. SAR is an active sensor that uses the reflections of signals beamed from a satellite towards the Earth’s surface—the “synthetic aperture” part comes from the radar using the satellite’s own motion to mimic a larger antenna, to capture reflected signals with relatively long wavelengths. There are dozens of governmental and commercial SAR satellites orbiting the planet, and many can be tasked to image new locations in a matter of hours. However, SAR imagery is still inherently two-dimensional, and can be even trickier to interpret than photographs. This is partly due to an effect called radar layover where undamaged buildings appear to be toppling towards the sensor. “Height is a super complex topic in itself,” says Michael Schmitt, a professor at the University of the Bundeswehr. “There are a million definitions of what height is, and turning a satellite image into a meaningful height in a meaningful world geometry is a very complicated endeavor.” Schmitt and his colleague Michael Reclastarted by sourcing SAR images for 51 cities from the TerraSAR-X satellite, a partnership between the public German Aerospace Center and the private contractor Airbus Defence and Space. The researchers then obtained high quality height maps for the same cities, mostly generated by lidar surveys but some by planes or drones carrying stereo cameras. The next step was to make a one-to-one, pixel-to-pixel mapping between the height maps and the SAR images on which they could train a deep neural network. The results were amazing, says Schmitt. “We trained our model purely on TerraSAR-X imagery but out of the box, it works quite well on imagery from other commercial satellites.” He says the model, which takes only minutes to run, can predict the height of buildings in SAR images with an accuracy of around three meters—the height of a single story in a typical building. That means the system should be able to spot almost every building across a city that has suffered significant damage. Pietro Milillo, a professor of geosensing systems engineering at the University of Houston, hopes to use Schmitt and Recla’s model in an ongoing NASA-funded project on earthquake recovery. “We can go from a map of building heights to a map of probability of collapse of buildings,” he says. Later this month, Milillo intends to validate his application by visiting the site of an earthquake in Morocco last year that killed over 2,900 people. But the AI model is still far from perfect, warns Schmitt. It struggles to accurately predict the height of skyscrapers and is biased towards North American and European cities. This is because many cities in developing nations did not have regular lidar mapping flights to provide representational training data. The longer the gap between the lidar flight and the SAR images, the more buildings would have been built or replaced, and the less reliable the model’s predictions. Even in richer countries, “we’re really dependent on the slow revisit cycles of governments flying lidar missions and making the data publicly available,” says Carl Pucci, founder of EO59, a Virginia Beach, Va.-based company specializing in SAR software. “It just sucks. Being able to produce 3D from SAR alone would be really be a revolution.” Schmitt says the SAR2Height model now incorporates data from 177 cities and is getting better all time. “We are very close to reconstructing actual building models from single SAR images,” he says. “But you have to keep in mind that our method will never be as accurate as classic stereo or lidar. It will always remain a form of best guess instead of high-precision measurement.” source: ieee
-
Satellite images analyzed by AI are emerging as a new tool in finding unmapped roads that bring environmental destruction to wilderness areas. James Cook University's Distinguished Professor Bill Laurance was co-author of a study analyzing the reliability of an automated approach to large-scale road mapping, using convolutional neural networks trained on road data, using satellite images. He said the Earth is experiencing an unprecedented wave of road building, with some 25 million kilometers of new paved roads expected by mid-century. "Roughly 90% of all road construction is occurring in developing nations including many tropical and subtropical regions of exceptional biodiversity. "By sharply increasing access to formerly remote natural areas, poorly regulated road development triggers dramatic increases in environmental disruption due to activities such as logging, mining and land clearing," said Professor Laurance. He said many roads in such regions, both legal and illegal, are unmapped, with road-mapping studies in the Brazilian Amazon, Asia-Pacific and elsewhere regularly finding up to 13 times more road length than reported in government or road databases. "Traditionally, road mapping meant tracing road features by hand, using satellite imagery. This is incredibly slow, making it almost impossible to stay on top of the global road tsunami," said Professor Laurance. The researchers trained three machine-learning models to automatically map road features from high-resolution satellite imagery covering rural, generally remote and often forested areas of Papua New Guinea, Indonesia and Malaysia. "This study shows the remarkable potential of AI for large-scale tasks like global road-mapping. We're not there yet, but we're making good progress," said Professor Laurance. "Proliferating roads are probably the most important direct threat to tropical forests globally. In a few more years, AI might give us the means to map and monitor roads across the world's most environmentally critical areas." journal: https://www.mdpi.com/2072-4292/16/5/839