- 0 replies
- 1,028 views
- Add Reply
- 0 replies
- 116 views
- Add Reply
- 1 reply
- 278 views
- Add Reply
- 0 replies
- 521 views
- Add Reply
- 1 reply
- 455 views
- Add Reply
Lyzenga Algorithm
By Agha,
Hello folks!
I am trying to use the Lyzenga Algorithm for estimating the depth of water in shallower areas, probably depths under 8-10 meters of lakes.
First of all, how accurate is this algorithm in practice?
Secondly, lets say i have the band values. can someone explain me how to retrieve those depths?
I am following the "Lyzenga Algorithm for Shallow Water Mapping Using Multispectral Sentinel-2 Imageries in Gili Noko Waters" paper, but there are 3 steps of getting NDWIs,
Lyzenga Algorithm for Shallow Water Mapping
By Agha,
Hello folks!
I am trying to use the Lyzenga Algorithm for estimating the depth of water in shallower areas, probably depths under 8-10 meters of lakes.
First of all, how accurate is this algorithm in practice?
Secondly, lets say i have the band values. can someone explain me how to retrieve those depths?
I am following the "Lyzenga Algorithm for Shallow Water Mapping Using Multispectral Sentinel-2 Imageries in Gili Noko Waters" paper, but there are 3 steps of getting NDWIs, NDCIs a
The Transformative Impact of GIS Mapping in Humanitarian Assistance
By IRES,
Understanding GIS Mapping
GIS Mapping is a technology and process used to capture, store, analyze, manage, and visualize geographic or spatial data. It combines geographical information such as locations and terrain features, with various types of data like environmental, social, economic, and demographic information, to create detailed and layered maps. These maps are powerful tools for understanding and interpreting spatial relationships, patterns, and trends.
Components of GI
TinyVQA, a compact multimodal visual question
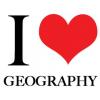
By Lurker,
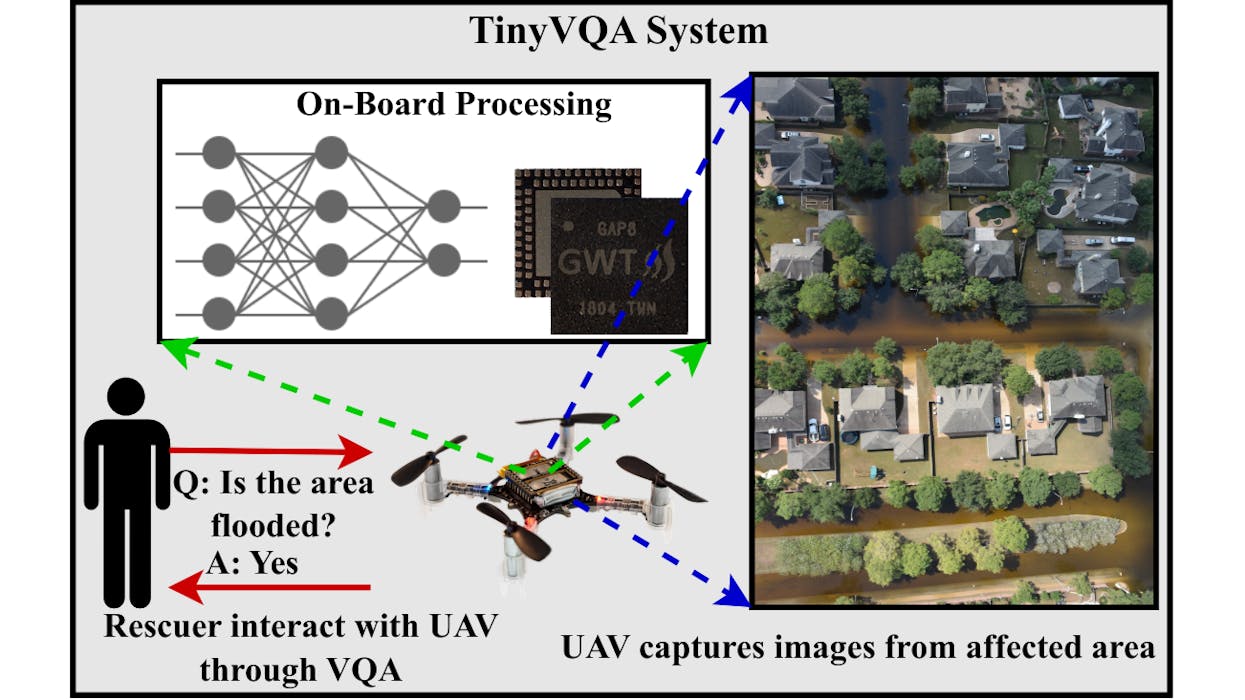
Multimodal machine learning models have been surging in popularity, marking a significant evolution in artificial intelligence (AI) research and development. These models, capable of processing and integrating data from multiple modalities such as text, images, and audio, are of great importance due to their ability to tackle complex real-world problems that traditional unimodal models struggle with. The fusion of diverse data types enables these models to extract richer insights, enhance decisi
AI Generates 3D City Maps From Single Radar Images
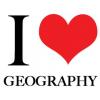
By Lurker,
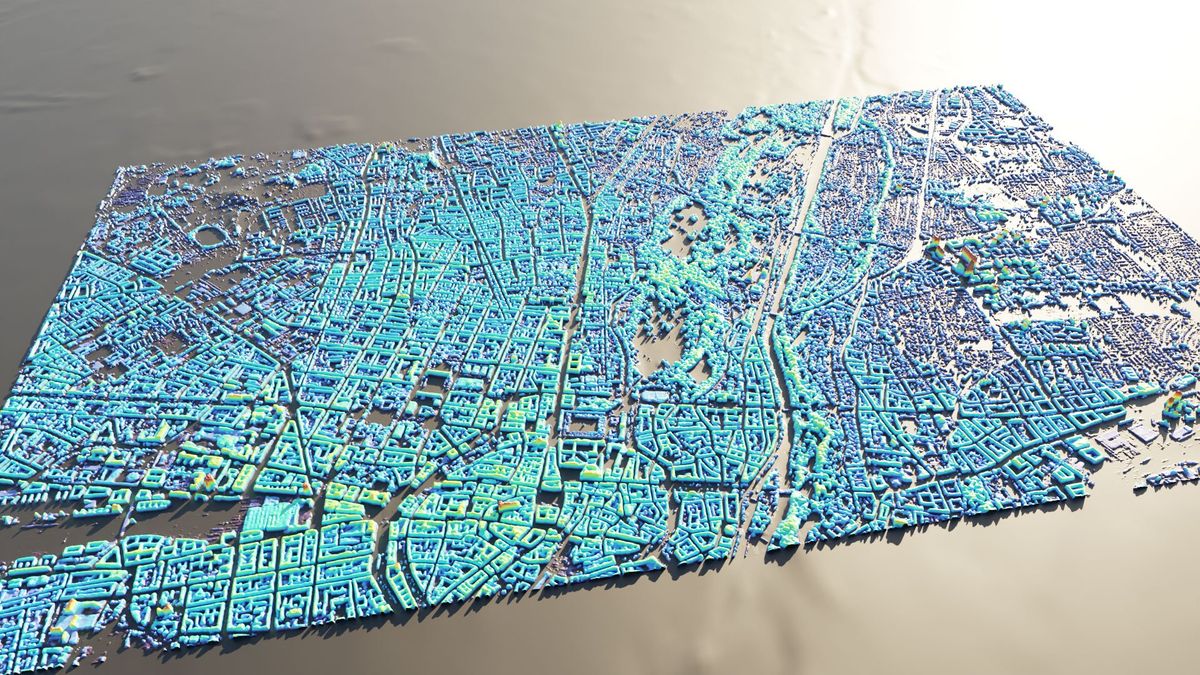
A new machine learning system can create height maps of urban environments from a single synthetic aperture radar (SAR) image, potentially accelerating disaster planning and response.
Aerospace engineers at the University of the Bundeswehr in Munich claim their SAR2Height framework is the first to provide complete—if not perfect—three-dimensional city maps from a single SAR satellite.
When an earthquake devastates a city, information can be in short supply. With basic services disrupte
-
Forum Statistics
8.7k
Total Topics43.2k
Total Posts